The insurance industry has always been data-driven, with actuaries and underwriters relying on statistical modeling to assess risks and price policies—but big changes are underway. Recent advances in artificial intelligence technology are opening up a whole new world of possibilities for this sector. From automated underwriting to claims processing and fraud detection, AI is being used to streamline processes, improve accuracy, and reduce operational costs.
In this article, we go over the most exciting ways that AI is transforming insurance. Keep reading for industry statistics, use cases, and inspiring case studies.
What is AI in insurance?
AI in insurance is an umbrella term that describes a wide range of AI-powered tools designed specifically for use in the insurance industry. It primarily includes specialized software that utilizes machine learning algorithms and natural language processing (NLP) technology to automate insurance processes including underwriting, claims processing, customer service, and more.
AI in insurance is a subcategory of insurtech, a broader term that describes technological products designed for the insurance industry.
7 use cases for AI in insurance
AI is revolutionizing both the way insurance companies function internally and how they service their clients. Below, we list the 7 most common applications of AI in insurance that are reshaping the industry.
1. Underwriting
Insurance underwriting has historically been a manual and time-consuming process. Underwriters have to collect and analyze customer information from various sources to decide whether insuring an individual or organization would pose a risk to the provider—and price policies accordingly.
However, no matter how thorough, human underwriters are likely to make high-stakes risk assessment mistakes or, on the other hand, limit eligible customers’ access to insurance based on personal bias.
With the right AI tools, insurance companies can reduce the underwriting process to minutes and virtually eliminate the risk of human error. Machine learning models can scan large and unstructured data sets in real time, providing issuers with a complete picture of a potential customer’s personal information, background, assets, claim history, existing insurance policies, etc. AI insurance solutions can then be used for predictive analysis, forecasting a potential client’s policy usage to match them with the most fitting insurance product.
2. Claims processing
Efficient claims management directly affects customer satisfaction and retention, shaping insurance companies’ bottom lines. In fact, according to a study by Accenture, claim processing times alone heavily impact how satisfied customers are with the overall handling of a claim:
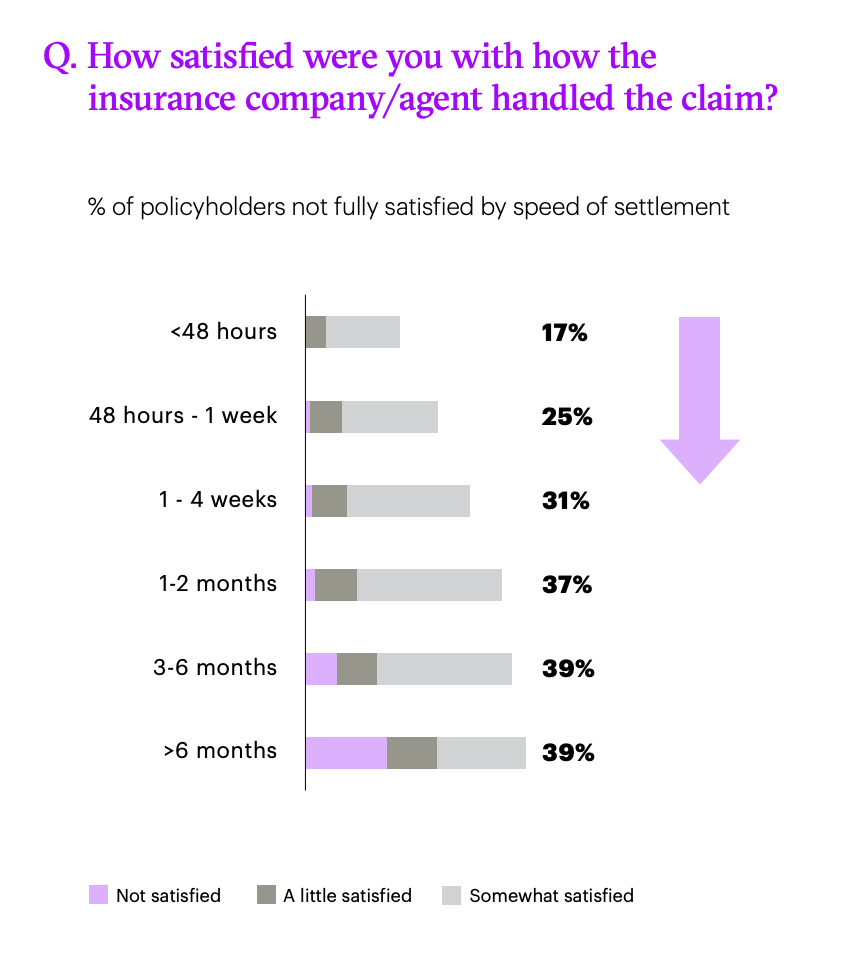
With that in mind, insurance companies need to do everything in their power to streamline the process of submitting insurance claims and reduce processing times.
AI technologies can be used to automate the following aspects of processing claims:
- Analyzing the contents of claims and supporting documents (including scans and photographs), and extracting relevant information
- Assessing claims and determining eligible coverage
- Prioritizing claims based on their urgency
- Identifying missing information or documents and requesting them from clients via automated emails or chatbots
- Detecting fraudulent claims
- Communicating claim status updates
3. Profit optimization
Machine learning tools have been successful in helping insurance companies optimize their pricing—and profits—by empowering teams to:
- Streamline data analysis
- Calculate customer lifetime value and profitability
- Uncover insights about customers’ expectations and flexibility in regards to pricing
- Run pricing experiments in live business environments (allowing for an immediate ROI)
Traditionally, insurance companies rely on individual underwriters to make one-off pricing decisions. With the help of machine learning, insurers can automate the pricing process and optimize premium calculations based on an analysis of relevant customer attributes.
Read our case study to see this process in action and find out how the team at Tribe AI partnered with an insurance provider to drive an overall premium lift of 12%.
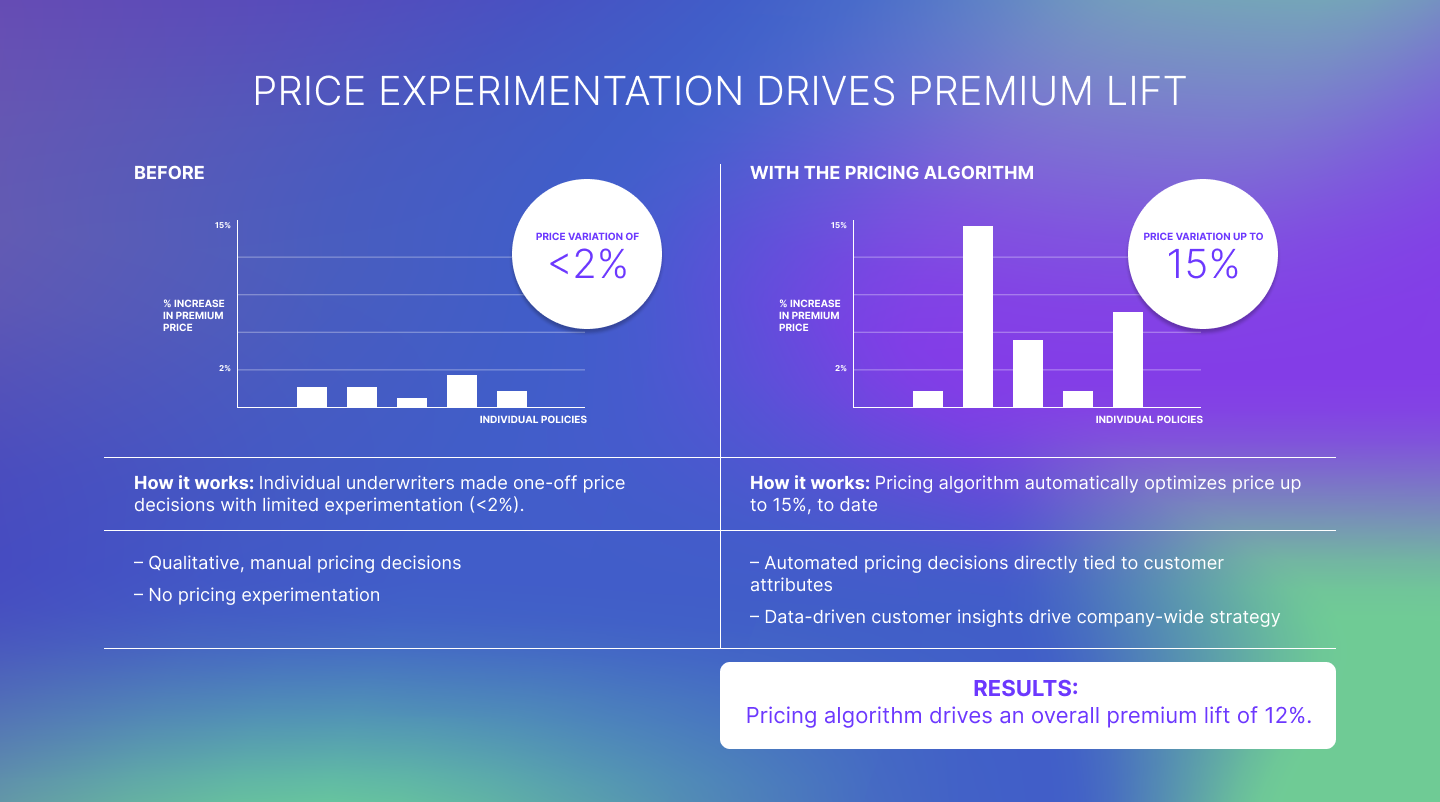
4. Product development
For years, competitive pricing and attractive discounts were the most important factors that determined how customers shopped for policies. According to a PWC study from 2021, that’s not necessarily the case anymore.
While pricing still does play a central role in informing product selection, consumers now value product variety and functional digital offerings over discounts.
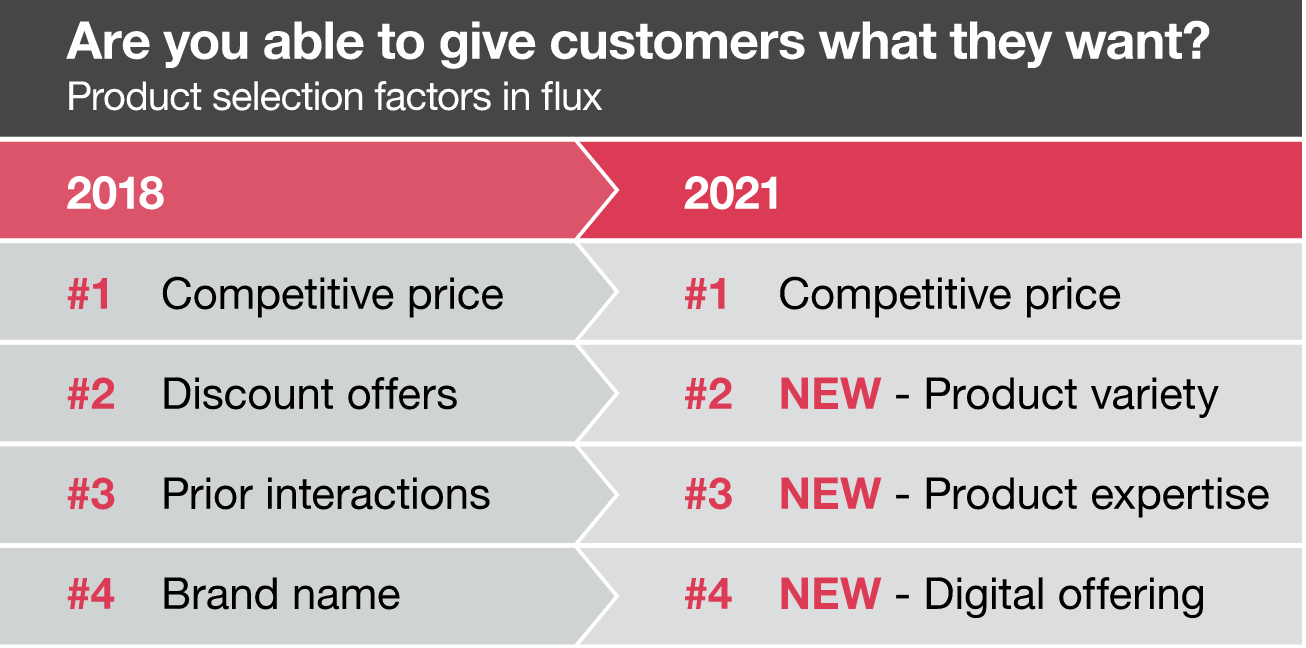
PWC also reports that between 2018 and 2021, the number of consumers who claimed they would switch carriers due to a lack of a user-friendly digital interface increased by 80%. In the same timeframe, 77% of respondents reported that they preferred to submit claims through their mobile devices.
These changing expectations are disrupting the industry. In order to stay competitive, insurance providers big and small are pushed to revise and innovate their product offerings.
From market research and segmentation to go-to-market promotion and implementation, product development can be a lengthy and costly process—but important parts of it can be automated. This is how artificial intelligence can streamline product development in insurance:
- Market research. AI algorithms can be used to collect and analyze consumer and competitive insights, and identify new R&D opportunities. This process might involve analyzing the sentiment of reviews and online mentions as well as reviewing market trends and tracking how they change over time.
- Product design. Using AI in the process of designing new products (e.g. mobile apps, online dashboards, automated claim processing flows) can save insurance companies money and empower them to deploy user-friendly and efficient products faster.
Personalization. AI can help insurance companies personalize elements of existing products based on insights regarding consumer preferences across regions and demographics.
5. Customer service
AI solutions based on natural language processing are changing customer service across industries. Insurance is no exception—and there are good reasons for insurance companies to be on board with innovation in the customer service space. According to a study by Accenture, $170 million in premiums is at risk within the next 5 years, industry-wide, due to policy-holders shopping around for a better customer experience.
Luckily, customer service tools powered by artificial intelligence are some of the easiest AI solutions to implement. Chatbots and virtual assistants can be used to answer customers' questions, guide them through the process of submitting claims, and route more complex inquiries to appropriate teams and human agents. They can also collect feedback, upsell insurance add-ons, and remind customers about upcoming renewal dates.
All of these applications make for a seamless overall customer experience and can greatly improve customer satisfaction and brand loyalty, ultimately resulting in higher retention rates.
6. Fraud detection
The FBI reports that the total cost of insurance fraud (excluding the healthcare industry) is estimated to be more than $40 billion per year. This affects both insurance companies and consumers—fraud costs the average family in the United States $400-$700 per year in increased premiums.
Easily scalable and capable of combing through vast amounts of data, artificial intelligence systems are much more effective than human controllers at detecting and preventing insurance fraud. Here are the areas of fraud detection that can be automated with AI:
- Analyzing data for patterns and anomalies that suggest fraudulent activity
- Predicting the likelihood of fraud based on risk factors, e.g. a customer’s claim history
- Identifying inconsistencies in claims and supporting documents
- Network analysis, i.e. scanning claims for signs of organized activity between individuals and/or organizations
- Alerting insurance providers and authorities of fraudulent activity in real-time
7. Risk management
In the United States alone, insurance companies collect over $1 trillion in premiums every year—and insurance fraud is not the only threat to these vast financial resources. Other common risk factors in the industry include underwriting errors, failed investments, cyberattacks, and natural catastrophes. While some of these are not preventable, smart risk management can mitigate the potential damage.
Here’s how AI can automate risk management in the insurance sector:
- Automated underwriting reduces the risk of human error that can result in selling underpriced insurance policies.
- Predictive modeling based on an analysis of patterns and trends in customer data helps insurance companies optimize pricing.
- Automated claims processing helps insurance providers recognize anomalies and flag potentially fraudulent claims at scale.
- AI-powered cybersecurity tools can strengthen data protection by detecting potential security threats in real time.
- Real-time reputation management via AI-powered social listening and sentiment analysis tools can help insurance companies avert PR crises.
Did you know?
US-based insurance company Lemonade “interviewed” ChatGPT, the popular conversational AI model, about how customers perceive insurance companies and what the future holds for AI in insurance. Check out the transcript here.
The benefits of AI for the insurance industry
As the insurance industry continues to embrace AI technologies, everyone will benefit: insurance companies as well as individual and business clients.
Streamlined business operations
Accenture reports that underwriters currently spend 40% of their time on non-core tasks (e.g. administrative work, compiling information, etc.). This inefficiency is estimated to cost the industry $85-$160 billion before 2027. Automating underwriting and pricing with AI will save insurance providers time—and big money.
Competitive edge
Regardless of whether you operate in the auto, home, business, life, or health insurance space, innovating your operations is bound to give you a competitive advantage. AI-powered tools will help you personalize your coverage plans, diversify your product offering, and offer better customer service.
Higher profits
AI-optimized pricing helps insurance companies turn higher profits while providing customers with personalized solutions and better customer experiences.
Reduced risk of human error
Human error is a risk factor at every stage of the insurance process. Underwriters often miss or misinterpret important information and inadequately price policies. Claim adjusters make mistakes regarding payouts, which can result in dissatisfied customers and lengthy appeal processes. Finally, customers make mistakes filing claims, compromising their outcomes. Handing these processes over to AI systems that can objectively analyze large data sets will minimize the risk of human error across the board.
Improved customer satisfaction
The use of AI technologies can help insurance providers improve the full lifecycle customer experience: from more personalized offerings, through faster and error-free digital claim processing, all the way to streamlined customer service and communications.
Glossary: AI technologies used in insurance
Machine learning
Machine learning (ML) is a branch of AI focused on creating systems and machines that learn from data. ML technology relies on artificial neural networks (ANNs) and simulated neural networks (SNNs) that mimic the structure of the human brain.
ML algorithms form predictions and make decisions based on patterns in the data they analyze. They are able to scan large, complex, and unstructured datasets in real-time, simplifying and speeding up decision-making processes for end users. Unlike traditional systems that only perform explicitly programmed functions, ML technology can autonomously produce infinite outcomes.
Deep learning
Deep learning is an advanced form of machine learning that allows modern AI algorithms to process images and even sounds rather than just text.
Natural language processing (NLP)
Natural language processing is a branch of AI that allows humans and “machines” (systems, devices, etc.) to interact using “natural language,” i.e. common vocabulary and conversational syntax rather than code or complicated commands. NLP algorithms can understand, interpret, and produce nuanced text.
NLP technology is most known for its use in chatbots and conversational AI tools like the popular Chat GPT — but possible applications go far beyond answering questions. Other popular uses of NLP include text classification and summarization, sentiment analysis, and named entity recognition (NER).
Read our case study to find out how Tribe AI helped three companies implement NLP solutions to improve the accuracy of their work, increase productivity, and gain a competitive advantage over competitors.
AI is the future of insurance: Become an early adopter
Artificial intelligence is helping insurance companies cut operational costs and optimize profits while improving the quality of their services and customer experience.
Don’t wait—the market rewards early adopters. Leveraging AI to innovate your business is not just profitable, it will also help you become a leader in your niche.
Not sure where to start? Our data science and machine learning experts are here to guide you all the way from ideation to implementation. Contact us to book a consultation and find out how AI can help your business.
AI in insurance FAQs
How is AI used in insurance?
AI in insurance is used to automate customer service, underwriting, pricing, processing claims, and analyzing large amounts of customer data to uncover valuable insights.
What is the benefit of AI in insurance?
The most common benefits of AI for insurance companies include savings that come from streamlined operations and higher profits made possible by AI-powered pricing algorithms. As the implementation of these new technologies becomes more popular in the insurance industry, customers can expect higher quality customer service, more personalized policies, and shorter claim processing times.
What is the role of AI in insurance pricing?
AI can be used to uncover customer insights, predict conversion behaviors, and empower insurance companies to optimize their pricing for greater profit. Read this Tribe AI case study to find out how our team of experts helped a leading insurance company implement ML-powered pricing algorithms that lead to a 12% overall premium lift.
Can AI replace insurance agents?
No. AI automates many workflows for insurance agents, including customer communications, data processing, and premium estimations. It also reduces the risk of human error. However, the technology can only reach its fullest potential when operated by skillful human professionals who know how to use the insights uncovered by artificial intelligence to provide personalized recommendations to their customers.