
The new poem-making machinery
The singularity, according to a poetry-writing AI.

Reddit Band ‘SFW’ deepfake community
How companies like Google and Reddit are dealing with more “complex” usages of advanced deep learning models.
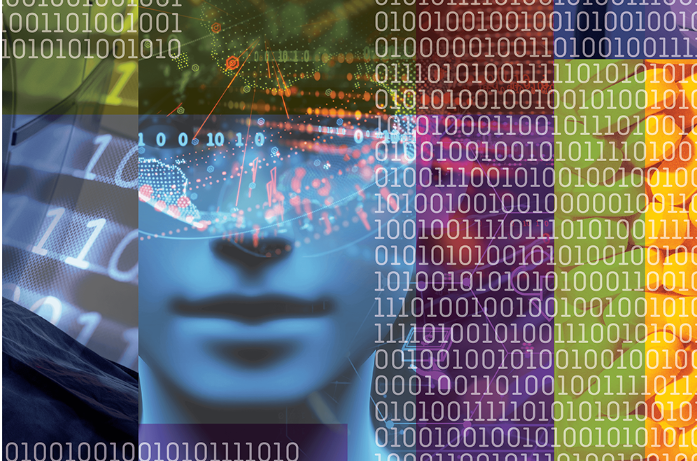
Smarter health: how AI is transforming healthcare
American health care is complex, expensive, and hard to access. This podcast series offers a good overview of the potential of AI to change that – from from predicting patient risk, to diagnostics, to just helping physicians make better decisions.

Emerging architectures for modern data infrastructure
An update on an article first published in 2020, article that explores data architectures across multiple contexts to help data teams stay on top of industry changes. The article not only explores current best-in-class stack across analytic and operational systems, but also what’s changed since 2020 and why.
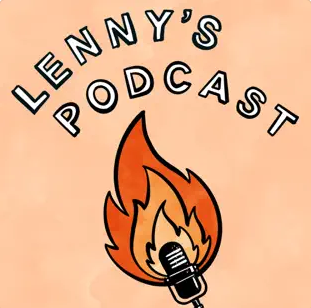
Gibson Biddle on his DHM product strategy framework, GEM roadmap prioritization framework, 5 Netflix strategy mini case studies, building a personal board of directors, and much more
An exploration of product strategy for consumer companies with former VP of Product at Netflix Gibson Biddle. Also covers recent events in the product world (e.g. Netflix and share price) and what PMs should know.

Synthetic data is about to transform artificial intelligence
According to a widely referenced Gartner study, 60% of all data used in the development of AI will be synthetic rather than real by 2024. What does that mean the modern economy, business operations, and applied AI?

The alignment problem
Published in 2020, this book examines what is know as the “alignment problem” in AI, from its technical foundations to its philosophical implications. The section on inverse reinforcement learning and how this might help build high-trust AI systems is particularly interesting.