ML Ops
Scale your AI systems with ML Ops
What is ML Ops?
ML Ops (Machine Learning Operations) combines machine learning with DevOps to streamline model deployment and management in production environments. By leveraging ML Ops, businesses can streamline ML processes, reduce costs, and accelerate time-to-market for their ML applications.
.png)
MLOps 101: A Primer on Machine Learning Operations
Get a comprehensive intro to MLOps, including practical recommendations for companies looking to implement MLOps practices and build a successful MLOps team.
Recent projects
Active
Built ML ops platform for public medical device company

+3
Active
Optimized deep learning pipelines for healthcare startup

+1
Active
Automated pricing strategy for a PE-owned insurance MGA
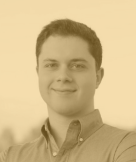
+3
Active
Optimized compute resources for public drug company
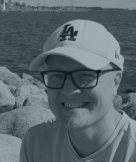
+4
How Tribe can help
ML infrastructure
MLOps can help companies set up robust ML infrastructure that is scalable and can support large-scale machine learning operations. This involves selecting appropriate hardware, recommending the use of TPUs, GPUs, or CPUs, and selecting the right cloud provider.
.png)
Model monitoring and management
MLOps helps companies monitor and manage machine learning models in production environments by tracking model and data versions, monitoring for data drift and uptime, and enabling rollback capability. It provides insights for diagnosing and debugging issues, and facilitates deployment into both cloud and IoT environments. By leveraging MLOps, companies can ensure that their models perform as expected, and make timely adjustments when issues arise.
.png)
Reproducibility & scalability
MLOps ensures scalable and reproducible machine learning models by implementing version control, logging, and testing processes, and setting up data and model versioning and data lineage. By leveraging MLOps, companies can develop and deploy machine learning models efficiently, while ensuring quality and consistency of outputs.
.png)
Security & compliance
MLOps ensures compliance with industry regulations and best practices for security by implementing appropriate data encryption, access controls, and testing procedures. By adhering to best practices like access control, encryption, and data anonymization, MLOps helps ensure compliance with data management regulations such as GDPR, HIPAA, etc. Companies can mitigate risks and develop and deploy machine learning models responsibly by leveraging MLOps for best practices in security and compliance.
.png)
Work with the best talent in AI

Oleksandr
PREVIOUSLY:
CTO at Togal
ML at Adblock
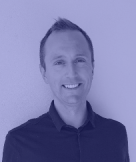
Rahul
PREVIOUSLY:
Director of Data Science at Appen,
Researcher at Toyota
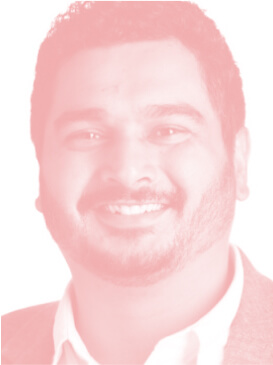
Alda
PREVIOUSLY:
Engineer at Cityblock Health
Sr Engineer Flatiron Health
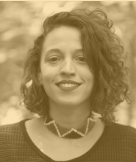
Scott
PREVIOUSLY:
Led ML at ClearBrain , Amplitude
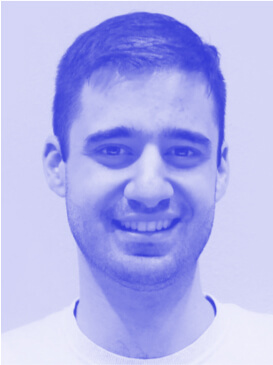
Catherine
PREVIOUSLY:
PhD from Cambridge University
Worked on NLP at Amazon Alexa
